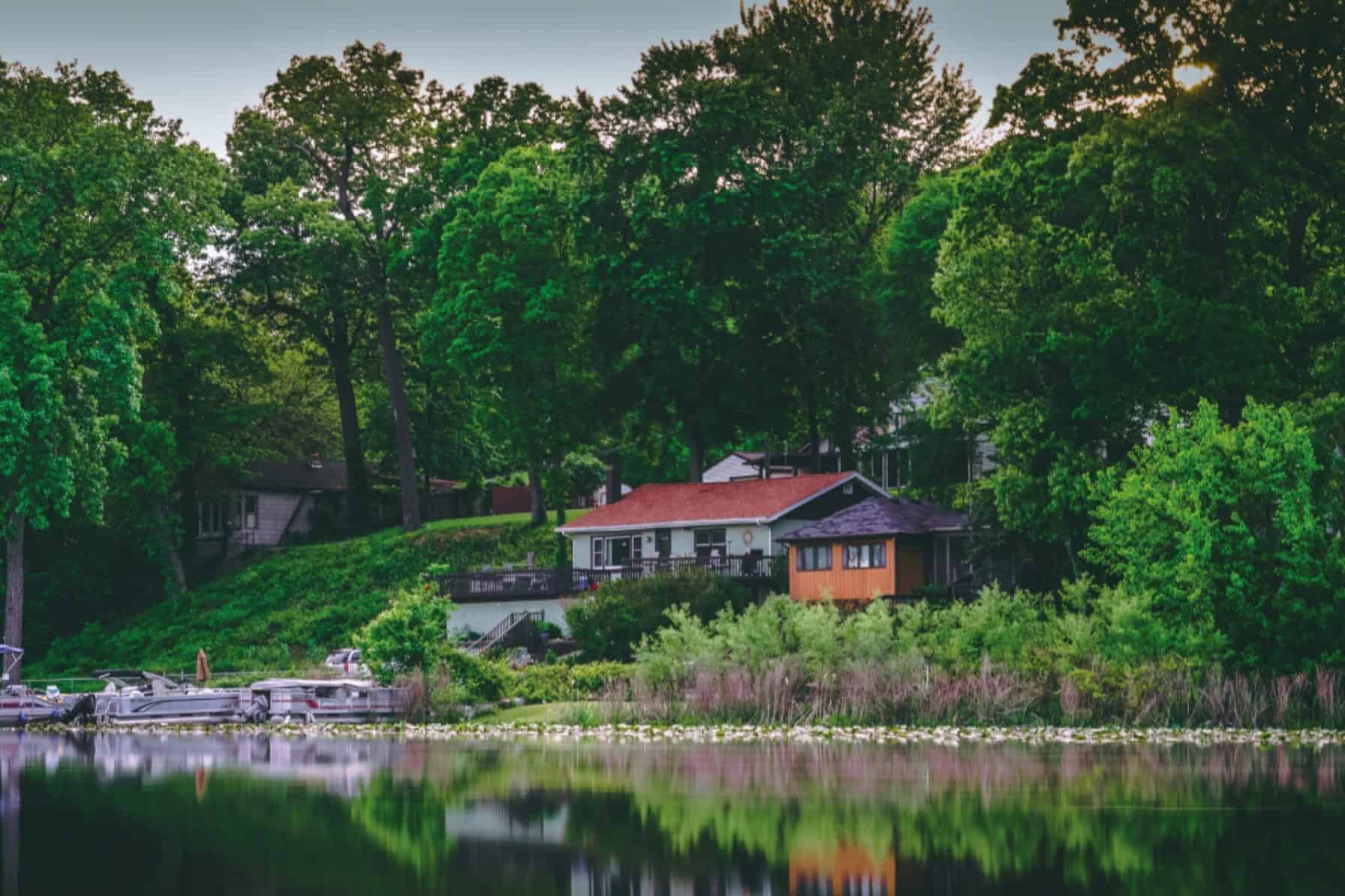
So we have moved beyond datalakes to data lakehouse, another iteration of collecting, storing and processing both structured and unstructured data. So how did we get here? Well before datalakes were popular, there were things known as data warehouses. These were typically enterprise-level data infrastructures, mainly on premise, which would store, process and analyse data in a structured, reliable, robust and consistent way.
This was perfect for large organisations as before the era of big data, they were the only ones who had large volumes of data and before the mass adoption of data science, they were mainly used for business intelligence.
Now one of the core reasons we moved to datalakes was because data warehouses were usually quite rigid and dealt predominantly with structured data. The business would usually decide what it wanted the information for, and then through usually bespoke ETL processes ingest the data before storing the data for business intelligence reporting and analytics which worked with the available data.
Then the era of big data emerged, with its associated discipline of data science, which meant that organisations were not only interested in structured data but also in unstructured data, such as emails, social media posts, images and even video footage. Data scientists were also far more inquisitive creatures, who were never happy with pre-processed structured data, but wanted access to the raw data, the data that came directly from production systems before it was ETL’d into databases and organised for efficiency, speed and accuracy.
To do this in a data warehouse posed two main problems. The primary being that data warehouses were not designed to store unstructured data. Data warehouses like their data structured, and in an organised schema, ready to be queried with a structured language, mainly SQL. The second was cost, keeping data in its raw format is expensive.
This new era of big data and the hunger of data scientists to explore different data sets in a single place led to the rise of datalakes. A place where different types of data could be ingested, from not just internal systems but also from external sources. This was facilitated by the use of APIs and other standard connectors and data formats. This meant that data could easily be ingested without a need for any development work. datalakes lived in the cloud natively and utilised cheap disk storage and elastic processing power.
Datalakes also allowed for common data querying tools to be easily integrated into them ranging from statistical, ML tools like R and Python to data visualisation tools such as Tableau and PowerBI.
However, as with all good things, there are some issues that present themselves to businesses. Firstly, for those wanting a structured database environment to analyse and run reports, a data warehouse was needed. That led to businesses having to run either two data platforms, others, however, decided to sit the data warehouse on top of the datalake so that once data was ingested, that data which was needed for business intelligence was ETL’d into a data warehouse.
Even with these workarounds, some critical components were not possible in a datalake which made many organisations uneasy about the legal, regulatory and compliance implications of the data they held in their datalakes. Not having full knowledge of what data they had in a datalake, the inability to apply some governance around it, the inability to catalogue their data and even having easy access became a challenge.
To solve this, solution providers are introducing data lakehouses. Operating on the cheap disk storage and open architecture of datalakes, but providing the structured organised approach of data warehouses, data lakehouses, will allow organisations to store whatever data they want, but with a level of governance, organisation and cataloguing of a data warehouse. Data lakehouses will also have tools that allow for the understanding of what data is stored in the data lakehouse and the ability for a range of people to analyse it with a variety of tools.
A data lakehouse essentially takes the good practice of a data warehouse, of having structure, using metadata, and indexing for speed, but with the scalability in terms of infrastructure and cost and the ability to query data with a variety of tools of a datalake.
So why doesn’t anybody want a data lakehouse? Well, it’s because most organisations will need a data lakehouse. Maintaining a data warehouse and datalake is proving to be costly and ungovernable. Meeting all the regulatory requirements of hosting, processing and storing data requires centralised infrastructure that cannot be easily done across two separate systems, nor can it be done in a datalake alone.
Those analysts who need to run regular reports will attest that, unless the data comes consistently in the same format every time, reports will break, and no matter how great the data pipelines, things change in the source systems which means errors emerge. So, datalakes for all their flexibility make for poor business intelligence solutions.
Most organisations would have been happy to continue with a datalake and not want a data lakehouse, but those serious about using data in their organisation will need to at some point think about getting a data lakehouse. Not only does it make sense when you want to do both data science and business intelligence in one system. But any organisation looking to deploy AI will find a need to move to a data lakehouse.
At Be Data Solutions, we help our clients define, develop and deliver data platforms, so drop us a line at hello@bedatasolutions.com and let’s have a chat about your data warehouse or datalake to see if you need a data lakehouse.