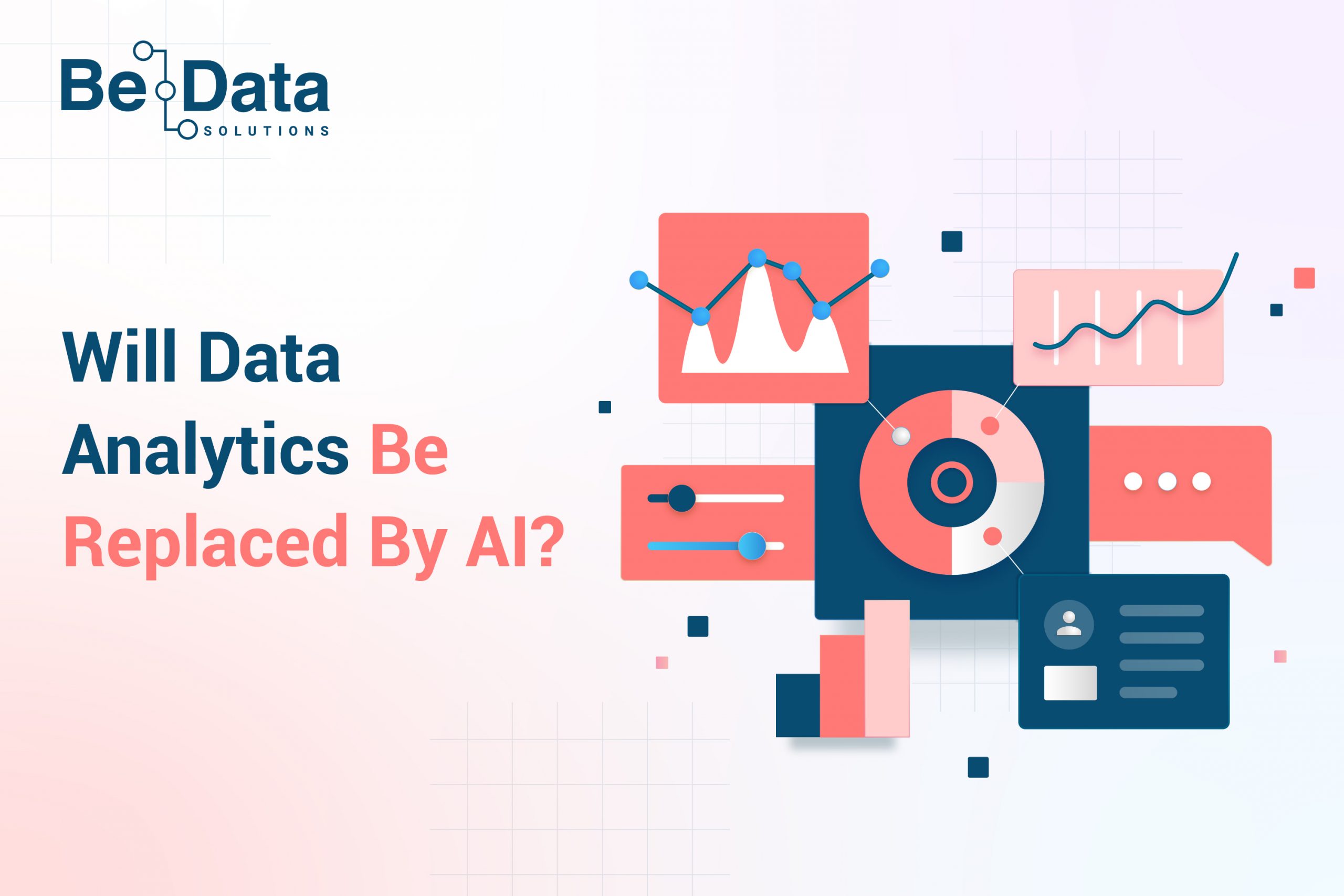
In the world of digital technology, data analytics has been a game-changer for businesses seeking to streamline operations and enhance decision-making. However, with the advent of artificial intelligence (AI), there is growing speculation about whether traditional approaches to data analysis will become outdated.
Will AI replace human analysts in deciphering complex datasets or will they work together in synergy? In this blog post, we explore the impact of AI on data analytics and examine how it is shaping the future of the industry.
The Impact of AI on Data Analytics: Will Traditional Approaches Be Replaced?
The emergence of AI has disrupted traditional approaches to data analytics, challenging businesses to rethink their strategies. With AI’s ability to process vast amounts of data at lightning speeds and detect complex patterns, it is no wonder that many see it as a potential replacement for human analysts. However, the truth is more nuanced than that.
While AI can certainly automate certain aspects of the analysis process and deliver insights quickly, it still requires human input in terms of designing algorithms and interpreting results. Moreover, there are some areas where human intuition and experience remain invaluable in identifying outliers or detecting errors.
Rather than replacing traditional approaches altogether, AI should be seen as a complementary tool that enhances the capabilities of data analytics. By working together with machine learning algorithms, humans can leverage technology to gain deeper insights into datasets that were previously impossible to analyse manually.
While there may be some overlap between AI and traditional approaches to data analytics, both have unique strengths that can be harnessed for greater efficiency and accuracy in decision-making.
The Future of Data Analytics: How AI is Shaping the Industry
The future of data analytics is exciting, and AI is at the forefront of shaping the industry. With its ability to analyse vast amounts of data quickly, accurately and efficiently, AI has revolutionised traditional approaches to data analysis.
AI-powered tools can now detect patterns in large datasets that humans might miss. This allows businesses to make informed decisions based on accurate insights in real-time. As a result, decision-making processes are becoming faster and more efficient.
In addition to improving efficiency, AI has also opened up new opportunities for innovation. With access to better technology comes a wealth of possibilities for businesses looking to expand their services or create new products.
However, with these advancements come challenges as well. The growing complexity of algorithms means that it’s increasingly difficult for human analysts to understand how they work and what biases they may contain.
Despite this challenge, there’s no doubt that AI will continue shaping the future of data analytics – ultimately driving greater efficiency and unlocking new business opportunities for those who embrace it.
AI vs. Data Analytics: Examining the Synergies and Distinctions
AI and data analytics are two distinct fields of study, yet they share many similarities. Both deal with large sets of data that require advanced computational skills to analyse and interpret.
At the core, data analytics is primarily concerned with extracting insights from historical data to inform future decision-making. In contrast, AI aims to replicate human intelligence by enabling machines to learn from experience and perform tasks without explicit instructions.
While both AI and data analytics can process vast amounts of information in real-time, they differ in their approach. Data analysts use predefined models or algorithms to identify patterns within existing datasets. Meanwhile, AI systems leverage machine learning techniques such as neural networks or deep learning algorithms that enable them to learn from new experiences and adapt over time.
The distinction between these two fields becomes more apparent when considering their applications. Data analytics typically focuses on descriptive or diagnostic analysis — explaining what has happened in the past or why it happened based on available evidence. On the other hand, AI is used for predictive modelling — forecasting future outcomes based on current trends.
AI-Powered Data Analytics: Revolutionising Insights and Decision-Making
AI-powered data analytics is transforming the way businesses collect, process, and analyse data. With the ability to handle large volumes of complex data sets at lightning-fast speeds, AI has made it possible for organisations to gain deeper insights into their customers’ needs and preferences.
By using machine learning algorithms to identify patterns in consumer behaviour, businesses can create personalised experiences that cater to individual customer needs. This not only improves customer satisfaction but also increases revenue by driving sales through targeted marketing efforts.
Moreover, AI-driven data analytics has streamlined decision-making processes by providing real-time insights into business operations. By monitoring key performance indicators (KPIs) such as sales figures, inventory levels, and production output rates in real-time, managers can quickly identify areas where improvements are needed and take corrective action immediately.
However, with great power comes great responsibility. The use of AI in data analytics raises ethical concerns regarding privacy rights and bias detection. As more companies rely on AI technology for decision-making purposes, there must be a concerted effort towards ensuring transparency and accountability in its usage.
The integration of AI-powered data analytics into business processes represents a significant step forward towards efficiency and innovation. However,it’s important to balance technological advancements with ethical considerations so that we continue harnessing its potential while mitigating any unintended consequences
Will AI Replace Data Analysts? Exploring the Changing Landscape
As AI technology advances, many people wonder whether it will eventually replace data analysts. While some fear that their jobs may become obsolete, the reality is more nuanced.
AI can certainly perform many tasks that were previously done by data analysts. For example, machine learning algorithms can quickly and accurately identify patterns in large datasets and generate insights based on those patterns. This means that AI can handle much of the routine work that was once done by human analysts.
However, there are still areas where human expertise is essential. Data analysts bring a wealth of domain knowledge and experience to the table, which allows them to interpret results in ways that machines cannot yet replicate. Additionally, they are able to ask questions about data sets and spot trends or anomalies that might not be immediately obvious.
Furthermore, even with advanced AI tools at their disposal, organisations need skilled professionals who can design experiments in order to collect meaningful data and optimise models accordingly.
While AI has the potential to automate many aspects of data analysis work – especially repetitive ones – it is unlikely to completely replace humans anytime soon. In fact, as businesses increasingly rely on big data for decision-making purposes across multiple sectors such as finance or healthcare among others then experienced individuals will remain integral parts of any organisation’s success in utilising these technologies effectively.
AI-Driven Data Analytics: Unleashing Opportunities and Challenges
AI-Driven Data Analytics: Unleashing Opportunities and Challenges
Artificial intelligence is revolutionising the way data analytics works. With AI-powered algorithms, large datasets can be analysed with more accuracy and speed than ever before. This can lead to new opportunities for businesses looking to gain insights into their operations.
One opportunity that AI-driven data analytics presents is the ability to identify patterns and trends in customer behaviour. By analysing large amounts of data from various sources, businesses can gain a better understanding of what their customers want and need, allowing them to tailor their products or services accordingly.
Another advantage of AI-driven data analytics is its ability to automate tasks that were once time-consuming and labour-intensive. For example, machine learning algorithms can analyse vast amounts of financial data in real-time, providing traders with valuable insights into market trends that would have taken hours or even days for humans to uncover.
However, along with these opportunities come challenges as well. One major challenge facing AI-driven data analytics is ensuring the accuracy and reliability of the results generated by machine learning models. As these models become more complex, it becomes increasingly difficult for humans to understand how they are making decisions.
Harnessing the Power of AI in Data Analytics: Advantages and Limitations
AI-powered data analytics is a game-changer in the industry, as it enables businesses to extract insights and make informed decisions quickly. By harnessing the power of AI, organisations can analyse vast amounts of complex data with ease and accuracy.
One significant advantage of AI in data analytics is its ability to process real-time data. With traditional approaches, analysing real-time information was difficult and time-consuming. However, AI algorithms can effectively handle massive sets of live streaming data that may be too cumbersome for humans or other software tools.
Another notable benefit is that AI-powered data analytics eliminates human biases while making sense of large volumes of complex information. Human analysts could sometimes miss critical patterns due to personal bias or cognitive limitations; however, using machine learning algorithms ensures unbiased analysis.
Despite these advantages, there are also limitations to consider when using AI in data analytics. For instance, the quality of output depends on the quality and quantity of inputted raw data. If there’s insufficient or inaccurate inputted raw material into an algorithmic model used for analysis purposes only then accurate conclusions cannot be drawn from such a system.
Ethical Considerations in AI-Enabled Data Analytics: Striking the Balance
As AI becomes increasingly integrated into data analytics, ethical considerations become more critical than ever before. One of the most significant concerns is privacy. As data analysts gather and analyse vast amounts of personal information, it’s crucial to ensure that individuals’ privacy is protected.
Another consideration is fairness and bias. AI-powered algorithms can unintentionally perpetuate biases in their decision-making processes based on historical data sets. Therefore, it’s essential to detect and address these biases to prevent unfair outcomes.
Transparency and accountability are also vital factors in ethical AI-enabled data analytics. Companies must be transparent about how they collect, store, and use gathered information from individuals while holding themselves accountable for any unethical practices or decisions identified by AI systems.
There is the concern regarding job displacement due to increased automation resulting from the integration of AI into data analytics processes. It’s important for companies to find a balance between utilising technology while still prioritising human intelligence and decision-making skills.
As we continue to integrate artificial intelligence into our daily lives through various applications like data analytics solutions; it’s necessary for businesses to always prioritise ethics alongside technological advancements in ensuring fair play at all times!
Conclusion
AI is not going to replace data analytics anytime soon. Instead, it will only enhance and revolutionise the way businesses analyse massive amounts of complex data for better insights and decision-making. The future of data analytics lies in its integration with AI, machine learning, and other advanced technologies that can help organisations turn their raw data into actionable intelligence.
While there are some concerns about the ethical implications of using AI-powered data analytics, these challenges can be addressed through responsible development and deployment practices that prioritise transparency, fairness, accountability, and privacy protection.