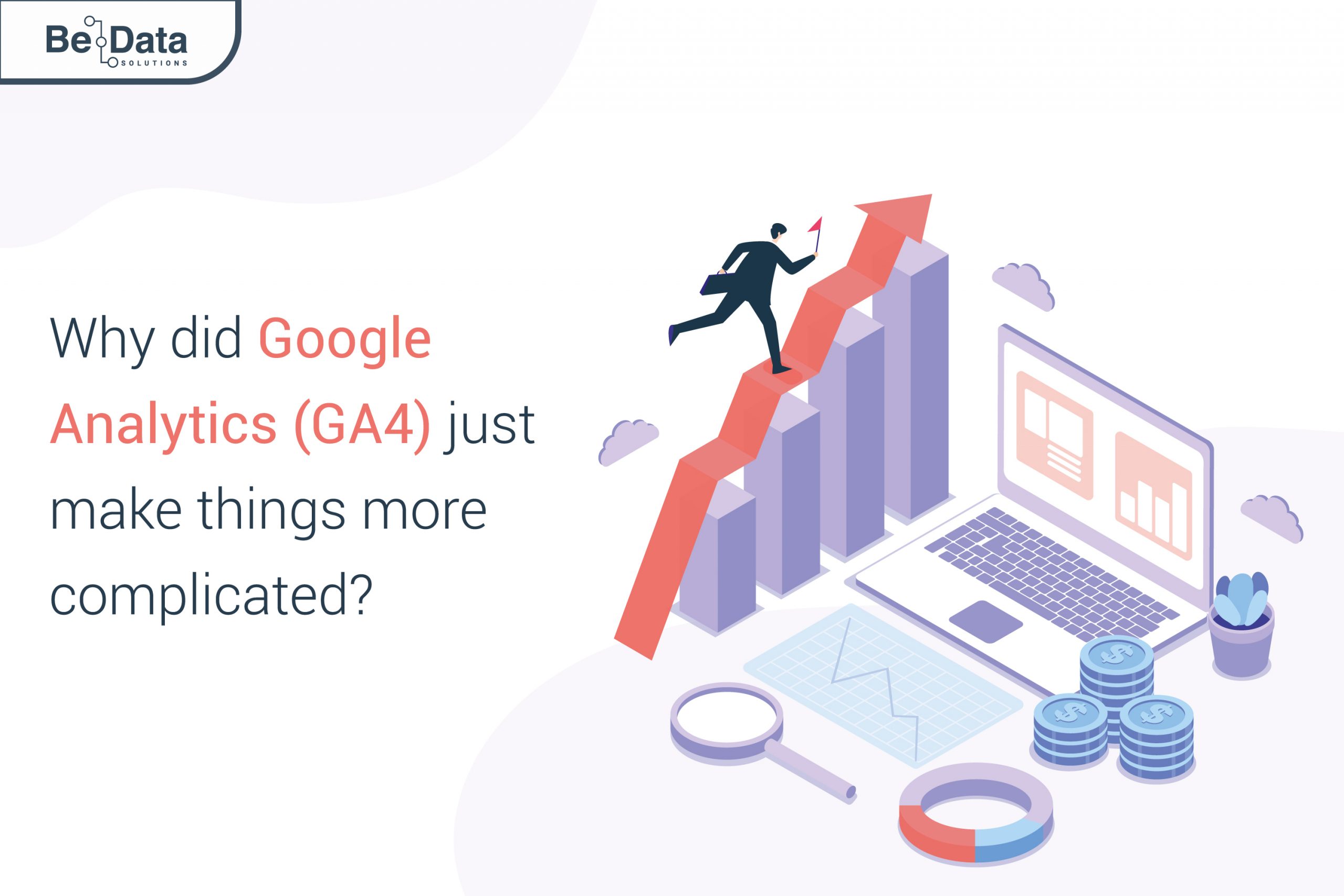
For many in the marketing space, whether client or agency side, life was relatively predictable with Google Analytics. Once you got over the continual challenge of maintaining your tracking so that you collected the data you needed, you could then easily analyse it in Google Analytics or simply connect LookerStudio (formerly Google Data Studio) and beautifully display that data in interactive dashboards.
Then Google announces that it will be sunsetting what is the current version of Google Analytics, referred to as GA3 or UA, for a new version of Google Analytics called GA4. On the surface, migrating to GA4 from UA seemed really simple. You just created a GA4 account in your Google Analytics console and for a while you could collect both UA and GA4 data in parallel.
If you are a seasoned digital marketer, you know that any changes in tracking will create some inconsistencies in numbers, and you just need to monitor these for a bit before you can calibrate your understanding of the performance of your digital properties. But with GA4, Google had introduced some significant changes.
Differences between UA and GA4
1. App tracking
GA4 has the ability to track website and app data in the same property. GA4 leverages the same measurement model as Google Analytics Firebase (used for mobile apps) where all interactions are captured as events. This new unified data schema between a website and a mobile app means that it will be much easier to combine data across them.
2. Event-Based Tracking
In UA, user interactions are tracked via sessions, so each interaction would be sent across a log file of preconfigured parameters. However, GA4 collects data at an event level, so those familiar with transactional data will understand the similarities. This means that reporting on that data is very flexible, as the event can be viewed as both a parameter and metric.
3. Bounce rate vs. engagement rate
GA4 no longer reports on bounce rate. Instead, Google has chosen to take a more “positive” approach and report on figures for engagement rate. Simply put, engagement rate is a ratio metric presented as a percentage, and the formula is engaged sessions/sessions.
What are engaged sessions you ask? These are sessions that lasted at least 10 seconds, had at least 1 conversion event, or had at least 2 page / screen views.
So in essence what Google has done is created an inverse metric for bounce rate. This will be a relief for those who had to explain that the one metric, bounce rate, in GA which when it went down, was a good thing, whilst others like users, visits, page view, time spent, etc., going up was a good thing. So now engagement rate aligns with the positive direction of all other metrics.
4. Predictive metrics
Google Analytics automatically enriches your data by bringing Google machine-learning expertise to bear on your dataset to predict the future behaviour of your users. With predictive metrics, you learn more about your customers just by collecting structured event data.
The three predictive metrics it has are;
- Purchase probability: The probability that a user who was active in the last 28 days will log a specific conversion event within the next 7 days.
- Churn probability: The probability that a user who was active on your app or site within the last 7 days will not be active within the next 7 days.
- Predicted revenue: The revenue expected from all purchase conversions within the next 28 days from a user who was active in the last 28 days.
As you use GA4 or are already you will notice other differences, some small like event tracking and others quite significant, like using AI to calculate users where privacy controls are in place.
Also read about: What’s All The Fuss With GA4?
But its not this that has made GA4 complicated
However, if you thought that differences in metrics or the way data is collected was your main issue, once you start using GA4 you will notice that the reporting interface is much leaner. There are very few predefined reports and even with a flexible report builder you can’t always get the report you need.
Even if you connect to Looker Studio, you will find that metrics and certain transformations that existed in UA are no longer available or not possible with GA4. Even if you extract to Google Sheets, you find that certain metrics and parameters are not possible to recreate.
This limitation in being able to build out these reports has been responded to by Google through the free integration of GA4 to BigQuery. This means that you can export all the data collected via GA4 into BigQuery and as a bonus you can store as much historical data as you like. To note, GA4 only allows you to store 14 months of historical data, and 2 months by default, which you must remember to extend to 14 months.
What is BigQuery?
Some of you may be asking what is BigQuery, whereas others may have heard of it, but not paid much heed as it was connected to Cloud and Big Data, something that was the domain of data engineers and data scientist, and something you didn’t have to worry about with the previous Google Analytics.
However, now you do need to worry about it, as if you want to create the reports and analysis you did previously and more, you need to know about BigQuery. Though Google have made its relatively simple, I use that term loosely, to connect GA4 to BigQuery using a connecter in your GA4 console, the setting up of BigQuery in Google Cloud Platform (GCP), setting up a billing account and then connecting it all, can be alien to many.
However that is what is needed to get the most out of GA4, even though it can be a daunting task, once done you will find that connecting LookerStudio to GA4 can make the analysis you run much more flexible, powerful and insightful.
But there is a catch, right?
Technically, there is no catch, you can store quite a bit of data in BigQuery, around 1TB and as long as your queries are reasonable, BigQuery has a free allowance. However, how do you know that you won’t incur spiralling costs? You’ve heard that the benefits of cloud computing is it’s pay as you go model, however, and if you’ve never had to use cloud computing then you are probably worried if you or your client, if you are a digital agency, is going to get a bill in the tens of dollars or thousands of dollars each month.
For that Google does offer a cost calculator for BigQuery/GCP, just Google it. However, unless you know what you are doing or going to be doing, it can be a bit overwhelming, choosing all the options and not knowing if that is what you need.
The other thing is that Google does allow you set budgets and alerts so that you don’t exceed your monthly allowance. You can either do a hard stop or Google can calculate the estimated monthly costs.
However, you are probably still a bit nervous about it all. For most of you, the Google Analytics you used was free, and the reports you provided your stakeholders had no additional costs. Now you need to go to your stakeholders, whether internal or external clients and ask for a budget to do something that was free before, and with some uncertainty if you have even estimated the correct amount and set the budget too low.
Should I even bother
For some it may be an opportunity to to just switch to another analytics provider, if you are going to pay, why not go with Adobe Analytics, or smaller players like Fathom Analytics, Matomo, Statcounter, Segment, etc., or even a free option like OpenWebAnalytics.
However, you shouldn’t quite yet close the coffin lid on GA4, if you can get past connecting to BigQuery, you are essentially on the journey to a cloud data warehouse, and with all the connectors Google Cloud Platform offers, you can connect all or most of your ad and martech to BigQuery, pulling in all your data into a single repository and unifying all the data from your digital marketing and advertising activity. As a cloud data warehouse, BigQuery can also connect to sources like your CRM system, your inventory systems and even your ERP systems.
Once all the data has been unified, possibilities open up, from running more insightful reports by combining disparate data sets, to a bit of data science. GCP has some powerful Machine Learning applications, which can be run on the data within BigQuery, allowing you to build predictive models, recommendation systems and customer/audience segmentations.
I need help
If you decide that climbing this mountain solo is not for you then get in touch with us. Our data engineers have been connecting GA4 to BigQuery, setting up a GCP instance and calculating your annual cost, if any, of running GA4 reports within BigQuery with Looker Studio for several of our clients.
Our data scientists have also gotten in on the act and built some very cool audience segmentation. One such machine learning application that they have built is the creation of “Publisher Unique Audiences (PUA)”. Publishers no longer need to sell bland audiences that others have, but can go to market with very unique audiences built from what visitors do wholly on the publisher website. These are unique to the publisher and are created purely from user behaviours on publisher owned sites, so no-one else can replicate them.
Get in touch with us if you need help with GA4, BigQuery, GCP or want to know more about the machine learning applications we are building on top of GA4 data.